Unlocking the Potential of Medical Datasets for Machine Learning
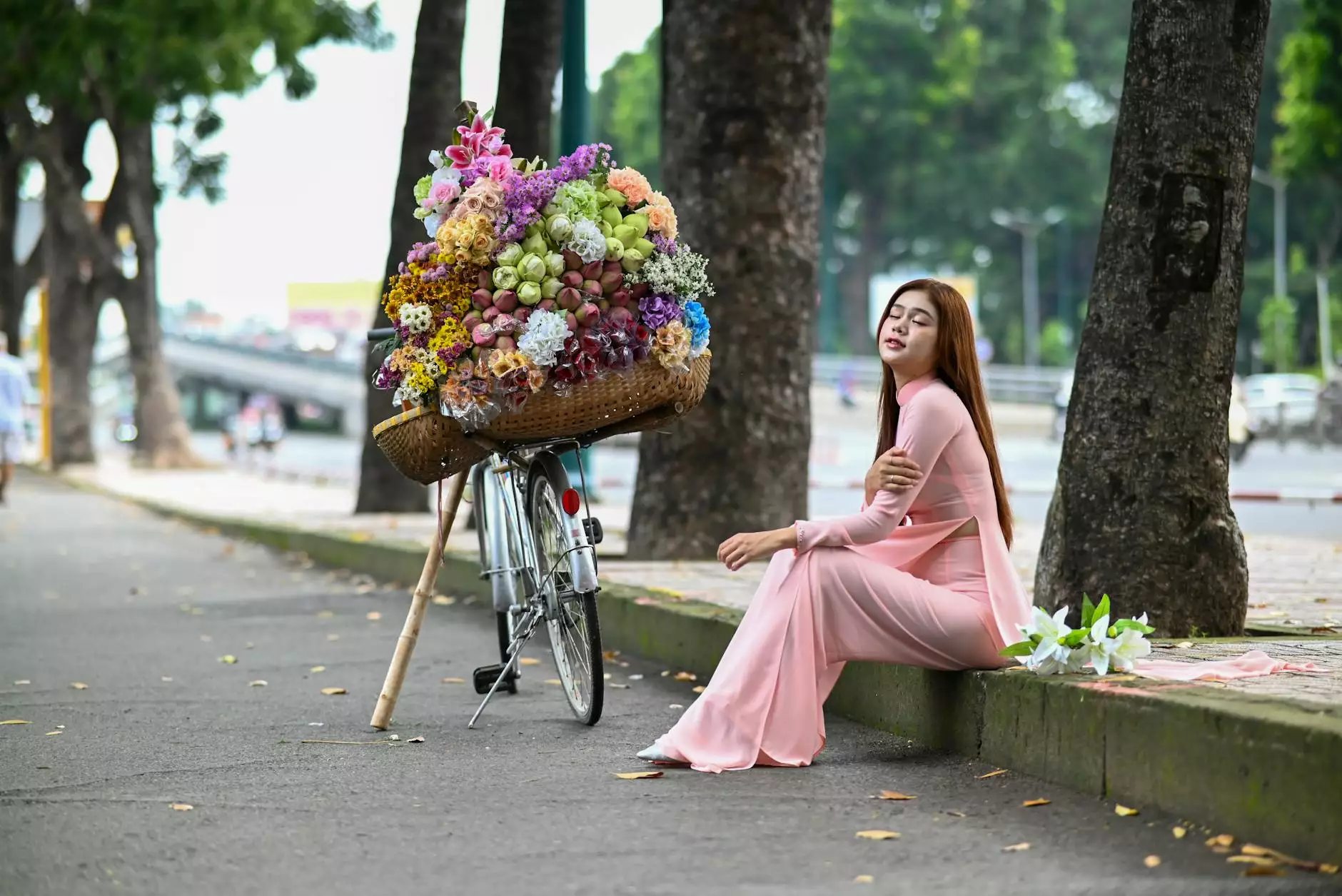
The intersection of medicine and technology has reached unprecedented levels, particularly with the advent of machine learning (ML). Central to this transformation is the availability and utilization of medical datasets for machine learning. These datasets are not just numbers and figures; they represent invaluable insights into patient care, treatment efficacy, and disease prediction and prevention.
The Importance of Medical Datasets
Medical datasets form the backbone of any machine learning project aimed at enhancing healthcare systems. By aggregating data from various sources, these datasets encapsulate extensive information that can be analyzed to derive meaningful patterns and predictions. Without these datasets, advancements in fields such as predictive analytics, drug discovery, and personalized medicine would be nearly impossible.
Types of Medical Datasets
There are several types of medical datasets utilized in machine learning, each serving a specific purpose:
- Clinical Trials Data: This data emerges from structured studies that assess the efficacy of new treatments under controlled conditions.
- Electronic Health Records (EHR): EHRs contain a wealth of patient data, including demographics, medications, allergies, and immunization status.
- Genomic Data: Sequencing data from various organisms, aiding in personalized medicine strategies based on genetic predisposition.
- Imaging Datasets: Large datasets of medical imaging (X-rays, MRIs, CT scans) which are crucial for training deep learning algorithms.
- Public Health Data: Aggregated health data made available by governmental and non-governmental organizations to track diseases and health trends.
How Machine Learning Transforms Healthcare
Machine learning algorithms analyze medical datasets to identify trends and make predictions that significantly improve patient care. Here are the key areas where machine learning is making waves:
1. Disease Prediction and Diagnosis
Machine learning techniques applied to medical datasets facilitate early disease prediction and diagnosis. By recognizing complex patterns in patient data, algorithms can help physicians predict the likelihood of diseases such as diabetes, heart conditions, and even cancer. With access to vast medical datasets, these predictive models learn from historical cases, improving their accuracy over time.
2. Personalized Treatment Plans
Machine learning also paves the way towards personalized medicine, where treatment strategies are tailored based on individual patient data. By analyzing the effectiveness of treatments administered to similar cases via medical datasets, healthcare providers can design customized treatment plans that optimize patient outcomes.
3. Drug Discovery
The drug discovery process has historically been cumbersome and costly. However, machine learning tools coupled with rich medical datasets enable researchers to simulate how new drugs interact with biological systems. These insights can dramatically shorten the time to market for new pharmaceuticals, ensuring that patients receive innovative treatments rapidly.
4. Operational Efficiency
By utilizing medical datasets for machine learning, healthcare facilities can enhance operational efficiency. Algorithms can be designed to predict patient admission rates, optimize resource allocation, and streamline workflows, ultimately improving the patient experience and reducing costs.
Challenges of Using Medical Datasets for Machine Learning
While the benefits are substantial, using medical datasets for machine learning comes with challenges:
- Data Privacy: Patient data is sensitive and must be handled with strict adherence to privacy regulations (e.g., HIPAA). Ensuring compliance and safeguarding data integrity is a significant concern.
- Data Quality: Incomplete or poorly organized datasets can lead to inaccurate models. Ensuring high data quality is paramount for successful machine learning outcomes.
- Interpretability: Many machine learning models, especially deep learning, operate as "black boxes." Ensuring that healthcare professionals understand model outputs can be challenging yet essential.
- Integration with Existing Systems: Incorporating new machine learning systems with existing healthcare infrastructure can pose logistical challenges.
The Future of Medical Datasets and Machine Learning
Looking ahead, the potential of medical datasets for machine learning remains vast:
1. Growing Availability of Data
With the rise of electronic health records, wearable technologies, and telemedicine, the availability of medical data is expected to increase exponentially. As this treasure trove of information continues to expand, the insights drawn from such datasets will become more profound and transformative.
2. Enhanced Collaboration
Collaboration among healthcare institutions, research organizations, and tech companies will likely foster the development of more robust datasets. Partnerships can lead to shared learning and breakthroughs in machine learning applications for healthcare.
3. Improved Regulatory Frameworks
As machine learning technologies evolve, so too will the regulatory environments. Future frameworks will likely place an emphasis on data privacy, security, and ethical model development, ensuring that patient welfare remains paramount.
4. Continuous Learning Systems
Advancements in artificial intelligence may lead to the creation of continuous learning systems, enabling real-time analysis of incoming data and refining predictive models as new patterns emerge.
Conclusion: Embracing the Future of Healthcare with Machine Learning
The integration of medical datasets for machine learning is not simply an option; it is becoming an essential component of modern healthcare. It promises to revolutionize how healthcare is delivered, making services more efficient, tailored, and effective. As we embrace this new frontier, collaboration and innovation will be key to overcoming challenges and maximizing the potential of machine learning in the health sector.
Keymakr.com is at the forefront of these innovations, exploring technologies and practices that optimize services across different sectors, including Home Services, Keys & Locksmiths, and beyond. By leveraging cutting-edge machine learning algorithms and comprehensive datasets, we contribute to the transformative landscape of healthcare and numerous other fields.
medical dataset for machine learning